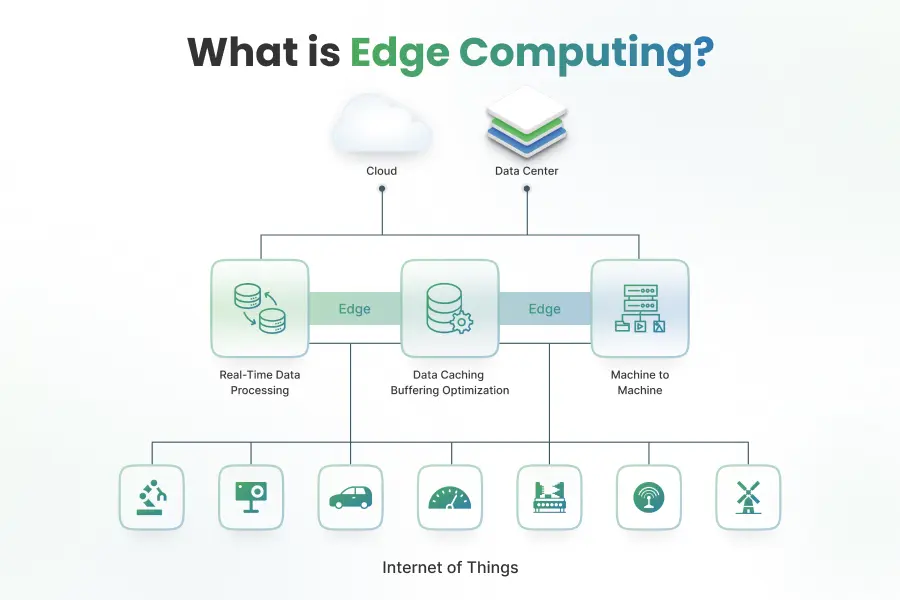
As today’s world becomes increasingly interconnected, the demand for faster, more efficient, and decentralized processing capabilities has given rise to the prominence of edge computing devices.
Here, in this guide, you can find edge computing explained and discussed, as well as the intricacies of edge device computing, from its fundamental principles to its real-world applications and the challenges it poses.
Edge Computing Definition
The edge computing definition is a disseminated computing pinnacle that performs data processing closer to the data generation source rather than relying on a centralized cloud server. Computing resources deploy at or near the “edge” of the network in edge computing, typically on devices like IoT (Internet of Things) devices, routers, gateways, or other local devices.
Edge computing solutions help reduce latency, enhance efficiency, and improve overall performance by processing data closer to where it is generated or needed rather than sending all data to a centralized data center for analysis.
This approach is particularly beneficial when real-time processing, low latency, and efficient bandwidth use are critical requirements. Edge computing complements cloud computing, allowing for a more decentralized and responsive edge device network architecture.
As per the markets and markets analysis, the global edge market is expected to grow from 53.6 billion in 2023 to USD 111.3 billion by 2028, at the Compound Annual Growth Rate (CAGR) of 15.7% during the forecast period.
How does edge computing work?
Edge computing operates on the principle of processing data near the source of its generation, reducing the need to send all data to a centralized cloud server. This decentralized approach improves efficiency, reduces latency, and is particularly advantageous in scenarios where real-time processing is critical. Here’s a breakdown of how edge computing works:
- Data Generation at the Edge: Typically, devices such as sensors, IoT devices, cameras, or other endpoints near the data generation area produce such data. Depending on the application, these devices capture information such as temperature, motion, images, or other relevant data.
- Perform Local Processing and Data Analysis: It involves running applications, algorithms, or scripts directly on these devices to extract meaningful insights or take immediate actions based on the incoming data. Local processing reduces the need to transmit large amounts of raw data to a central server.
- Filter and Prioritize Data Based on Predefined Rules or Conditions: It enables the system to focus on transmitting only relevant or critical information to the central cloud or data center, optimizing bandwidth usage and minimizing the impact on the network.
- Maintains a Connection to Centralized Cloud Services, Emphasizing Local Processing: This connection is helpful for tasks that require additional computational resources, storage, or long-term analytics. Edge devices can send aggregated data, updates, or insights to the cloud, ensuring a balance between local and centralized processing.
- Adopts Decentralized Architecture where computing resources are distributed across the network, closer to the data sources. It stands in contrast to traditional cloud computing, as in that process, data is sent to centralized data centers for processing. The decentralized edge computing approach contributes to scalability, flexibility, and resilience.
Why is edge computing important for OEMs?
Edge computing is crucial because it can process data nearer to the source, reducing latency and enhancing real-time applications. Minimizing data transmission to the cloud optimizes bandwidth and ensures efficient use of resources. The decentralized nature of edge computing enhances privacy and security by processing sensitive data locally, reducing the risk of breaches.
Scalability is improved through distributed processing, enabling seamless integration with many devices. Edge computing supports offline operation, making it invaluable in scenarios with unreliable connectivity.
Real-time decision-making, redundancy, and cost efficiency are additional benefits, contributing to its significance in various industries. As a foundational technology for emerging trends like 5G and edge computing IoT, edge computing helps shape the future of digital ecosystems.
How Edge Computing Differs from Cloud Computing?
Aspect | Edge Computing | Cloud Computing |
Location of Data Processing | Near the source of data generation (at the edge) | Centralized servers in data centers |
Latency | Low latency due to local processing | May introduce higher latency as data travels to and from central servers |
Use Case Focus | Real-time applications (e.g., IoT, AR, autonomous vehicles) | Versatile applications, including storage, data processing, and non-real-time applications |
Bandwidth Usage | Optimizes bandwidth by processing data locally | Data transfer to centralized servers may lead to higher bandwidth usage |
Scalability | Inherent scalability through distributed resources | Achieved scalability through provisioning resources in central data centers |
Data Privacy and Security | Enhanced data privacy by processing sensitive information locally | Implemented edge device security measures, but concerns about transmitting sensitive data to the cloud |
Reliability and Redundancy | Increased reliability and redundancy with distributed processing | Redundancy and failover mechanisms in centralized data centers, potential vulnerabilities due to centralized location |
12 major benefits of incorporating edge computing
Edge computing offers various benefits, making it a compelling and strategic choice for various applications and industries. Here is a comprehensive list of the key advantages of edge computing.
1. Optimizes Bandwidth Usage by Processing Data Locally
Only relevant or critical information is transmitted to centralized servers, reducing the need to send large amounts of raw data over the network. It is particularly beneficial in scenarios where bandwidth is limited or costly.
2. Reduces Latency by processing data nearer to the source
It is crucial for applications requiring real-time responses, such as autonomous vehicles, augmented reality, and industrial automation, enhancing user experience and system responsiveness.
3. Enables Real-Time Data Processing and analysis
It is essential for applications like video analytics, monitoring, and control systems where immediate insights and responses are required. Localized processing contributes to faster decision-making.
4. Processing sensitive data locally enhances privacy and security
Edge computing decreases the need to transmit sensitive information over external networks, mitigating the risks associated with data transmission. It is essential in industries like healthcare and finance, where data privacy is a top priority.
5. Inherent Scalability by distributing computing resources across the network
Adding New edge devices can quickly expand the computing capacity. This distributed architecture provides flexibility in adapting to changing computational needs.
6. Allow devices to operate offline or with limited connectivity
It is beneficial in environments where there is no guarantee for continuous edge device network connectivity. Edge devices can perform essential processing tasks independently, ensuring operational continuity.
7. The distributed nature of edge computing improves reliability and redundancy
Even if connectivity to the central cloud is lost, localized processing can continue to operate, reducing the impact of network disruptions. It is imperative in critical applications where downtime is not acceptable.
8. Optimizes computing resources by distributing processing tasks across devices
This results in more efficient resource utilization, as tasks are performed closer to where the data is generated, reducing the need for centralized processing for every operation.
9. Support the potential of the Internet of Things (IoT)
Edge Computing allows for efficient handling of the massive value of data generated by IoT devices and supports the low-latency requirements of 5G applications.
10. Imperative in various Industry-Specific Applications
Edge computing is essential in various industries, including healthcare, manufacturing, retail, and smart cities. It enables tailored solutions for specific industry challenges, providing customized and efficient computing solutions for diverse applications.
11. Reduces extensive cloud infrastructure needs and data transfer costs
Edge computing can help save costs by diminishing the need for extensive cloud infrastructure and decreasing data transfer costs. Local processing at the edge devices eliminates the constant back-and-forth data transmission to centralized servers.
12. Provide an Enhanced User Experience
Applications that leverage edge computing often provide a better user experience due to reduced latency and faster response times. It is particularly relevant in scenarios where instant feedback or interaction is crucial, such as gaming, virtual reality, and video streaming.
Challenges of edge computing and opportunities
Challenge | Opportunity | |
Security Concerns | Distributing computing resources across a network raises data and edge device security concerns. Edge devices may be more vulnerable to cyber and physical threats. | Develop robust security protocols and encryption mechanisms specific to edge computing. Execute security best practices to protect data and devices at the edge. |
Device Heterogeneity | Edge computing involves diverse devices with varying capabilities and architectures. Managing this heterogeneity can be challenging for developers and system administrators. | Standardize protocols and interfaces to ensure interoperability among different edge devices. Develop tools and frameworks that abstract device heterogeneity for ease of development. |
Data Management | Edge devices generate large volumes of data, and managing, processing, and storing this data can be complex. | Implement efficient data filtering and aggregation at the edge to minimize the data sent to the cloud. Leverage edge analytics to process and analyze data locally, reducing the need for centralized storage. |
Scalability | Scaling edge computing solutions can be challenging due to the distributed nature of the architecture. | Design scalable edge architectures to accommodate new devices easily. Implement edge orchestration tools to manage and scale edge deployments efficiently. |
Intermittent Connectivity | Edge devices may operate in environments with intermittent or unreliable connectivity, posing challenges for real-time data processing. | Design edge applications to operate efficiently in offline or low-connectivity scenarios. Use edge caching mechanisms to store critical data locally for immediate processing. |
Standardization and Compatibility | Lack of industry-wide standards for edge computing may lead to compatibility issues between devices and platforms. | Advocate for and contribute to developing industry standards for edge computing. Standardization can improve interoperability and facilitate broader adoption. |
Resource Constraints | Edge devices often have limited computational resources, including processing power, memory, and storage. | Optimize edge applications for resource efficiency. Explore advancements in edge hardware to improve the capabilities of devices, allowing for more complex computations at the edge. |
Deployment and Management | Deploying and managing a large number of distributed edge devices can be complicated and resource-intensive. | Develop user-friendly edge device management tools and platforms for deploying, monitoring, and updating edge devices. Automation and remote management capabilities can streamline these processes. |
Top Edge Computing Applications in 2024
Edge computing applications span across various industries, leveraging the benefits of localized data processing, low latency, and real-time decision-making. Here are notable examples of edge computing applications:
1) Industrial Internet of Things (IIoT)
In manufacturing and industrial IoT settings, edge computing is applied for real-time monitoring, predictive maintenance, and machinery control. Sensors and devices on the factory floor process data locally, optimizing production efficiency.
2) Energy Grid Optimization
In the energy sector, edge computing is applied to optimize the diffusion and consumption of energy. Localized data processing from sensors and smart meters helps improve grid efficiency and reliability.
3) Edge AI in Surveillance Systems
Surveillance systems benefit from edge computing by enabling real-time video analytics. Local processing of video feeds allows for object detection, facial recognition, and immediate response to security events.
4) Asset Tracking in Logistics
Edge computing is utilized for real-time asset tracking in logistics and supply chain management. Sensors and RFID tags provide accurate location data, and edge devices process this information locally for efficient inventory management and route optimization.
5) Edge Analytics for Process Optimization
Edge computing supports analytics for real-time process optimization in various industries. Data from sensors and devices are analyzed locally, allowing for quick identification of patterns, anomalies, and opportunities for improvement.
Best Platform Use Cases of Edge Computing for Industrial IoT
The best use of an edge computing platform for IoT (Internet of Things) depends on the specific requirements of the IoT application and the industry it serves. However, some common and impactful use cases where edge computing platforms excel in supporting IoT deployments include:
Real-Time Data Processing:
Use Case: It is ideal for applications that require real-time data processing, such as monitoring and control systems. Edge Computing Examples include real-time sensor data analysis in industrial environments, smart buildings, or healthcare monitoring.
Predictive Maintenance:
Use Case: Edge computing platforms enable predictive maintenance by processing data from IoT sensors locally. It allows for early detection of equipment anomalies, decreasing downtime and maintenance costs in industries like manufacturing, transportation, and energy.
Video Analytics and Surveillance:
Use Case: Edge computing enhances video analytics in surveillance systems by processing video feeds locally. Object detection, facial recognition, and other analytics can be performed in real-time, improving security and reducing the need for transmitting large amounts of video data to a central server.
Connected Vehicles and Transportation:
Use Case: Edge computing is crucial for connected vehicles and smart transportation systems. It enables real-time processing of data from sensors in vehicles, allowing for applications like traffic management, collision avoidance, and vehicle-to-vehicle communication.
Environmental Monitoring:
Use Case: Edge computing is valuable for environmental monitoring IoT applications, such as air quality monitoring, water quality analysis, and weather stations. Local processing facilitates real-time analysis and immediate responses to changing environmental conditions.
Conclusion
In conclusion, edge computing stands at the forefront of transformative technological advancements, reshaping the landscape of data processing and application deployment. Its ability to bring computation closer to the data source, reducing latency, and enabling real-time decision-making will make the Edge Computing Industry Grow Substantially at A CAGR of 30% during 2023-28.
This robust growth underscores the immense opportunities that lie ahead for organizations embracing Flex83, propelling them toward a future defined by innovation, agility, and sustained success in the digital era.
From enhancing the capabilities of Internet of Things (IoT) devices to revolutionizing industries, you can harness the power of Flex83. It emerges as a pivotal AEP platform for business transformation, offering a seamless bridge from legacy systems to modern business models. Also, it can unlock the potential to build intelligent products, enhance operational efficiency, and position yourself at the forefront of the rapidly evolving business landscape.
FAQs
Q1: What is edge computing, and how does it differ from traditional cloud computing?
A: Edge computing is an extension of cloud computing that brings computation closer to the data source. Unlike traditional cloud computing, which centralizes processing in data centers, edge computing performs computations on devices or edge servers, reducing latency and improving real-time processing capabilities.
Q2: What is an edge computing platform, and how is it used?
A: An edge computing platform is a set of technologies and services that facilitate the deployment, management, and scaling of applications at the edge of the network. It includes tools for developing, deploying, and orchestrating edge applications, making it easier to leverage the benefits of edge computing.
Q3: Can you provide edge computing examples of devices?
A: Edge computing devices include routers, gateways, IoT devices, edge servers, and smartphones. These devices have embedded processing capabilities, allowing them to perform local computations and enhance the efficiency of applications.
Q4: What is the architecture of edge computing?
A: Edge computing architecture is decentralized and distributed. It involves edge devices that process data locally, reducing the need for centralized processing in the cloud. The architecture includes edge nodes, gateways, and cloud connections, forming a network that optimizes data processing.
Q5: How does Mobile Edge Computing (MEC) relate to 5G technology?
A: Mobile Edge Computing (MEC) is a key component of 5G networks. It brings computation capabilities to the edge of the mobile network, reducing latency and supporting real-time applications. MEC is essential for delivering the high-speed, low-latency requirements of 5G technology.
Q6: What are some common applications of edge computing?
A: Edge computing applications span various industries, including autonomous vehicles, industrial IoT, smart cities, healthcare monitoring, retail analytics, and more. Its ability to process data locally enables real-time decision-making in these diverse applications.
Q7: How is security addressed in edge computing environments?
A: Edge computing enhances security by processing sensitive data locally, reducing the risk of transmitting it over external networks. Encryption, access controls, and secure communication protocols are implemented at the edge to safeguard data throughout its lifecycle.
Q8: Does AWS use edge computing in its services?
A: Yes! AWS (Amazon Web Services) offers services and solutions that leverage edge computing. AWS Wavelength, for example, brings AWS services to the edge of the 5G network, allowing developers to build ultra-low-latency applications for end-users.
Nishant Puri, CISO at IoT83
Nishant carries professional expertise in team collaboration and network security solutions. He excels at aligning the needs of key business stakeholders, including Sales, Marketing, and Product Engineering, with pragmatic and efficient approaches that meet both short-term and long-term strategic goals.
Before joining IoT83, Nishant held a leadership position at Cisco America Partners, where he led sales and technology solutions. He was also a frequent speaker for Cisco APO, showcasing his knowledge and experience in the field. Being a Cisco-certified Inter-Networking Expert in Security and Collaboration, Nishant brings a wealth of technical expertise to his role. He is also inclined to identify digital discontinuities and is adept at mapping out effective digital transformations.